Mary Hall Elected Vice Chair of Computing Research Association Board of Directors
Mary Hall Elected Vice Chair of Computing Research Association Board of Directors
Goldman Sachs U of U Former Intern Panel - April 3
Event Details
April 3, 2025
5:00 PM- 6:00 PM
Warnock Enginerring Building (WEB) room 1250
Please join us for the U of U Former Intern Panel. This event will provide you an opportunity to learn more about our businesses, network with Goldman Sachs professionals and learn more about ourSummer program opportunities with Goldman Sachs.
This event is open to all sophomore engineering students as well as incoming new analysts and summer analysts.
Goldman Sachs is where exceptional people build extraordinary careers. We hire people with diverse skill sets, interests, and backgrounds – and we provide them with the hands-on experience to business challenges and opportunities to learn firsthand from the very best.
If you are someone who thrives on excellence, join us at our upcoming event to learn more about Goldman Sachs and our long-standing apprenticeship culture.
We look forward to meeting you.
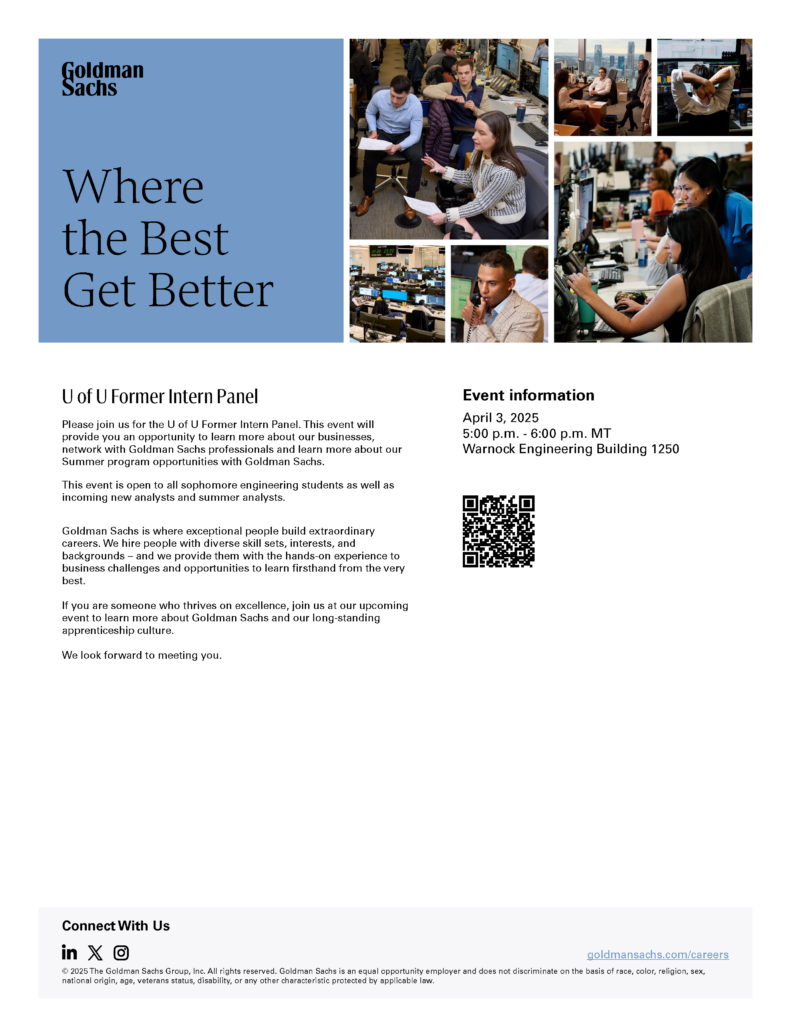
NSF-Simons CosmicAI Institute Seminar Series with Varun Shankar - March 26
Event Information
March 26, 2025
12:00 PM – 1:00 PM
Evans Conference Room, Warnock Engineering Building (WEB) Room 3780
Zoom Access
Meeting ID: 958 8067 9001
Passcode: 255836
Structure-Preserving, Low-Parameter, Interpretable, Operator Learning for Surrogate Modeling with Varun Shankar (Assistant Professor, Kahlert School of Computing)
Scientific machine learning (SciML) is a relatively new scientific discipline that weds scientific computing and high performance computing with carefully designed machine learning (ML) techniques. In the context of astrophysics, SciML has been applied to galaxy classification and identification, outlier detection, and uncertainty quantification.
Within SciML, operator learning is a rapidly emerging and powerful new paradigm for surrogate modeling across engineering and the sciences, with recent successes in climate modeling, material design, and carbon sequestration problems (to name a few). In this talk, I will present a unified framework that encompasses many operator learning paradigms and use this to present three advancements in operator learning: (1) the Kernel Neural Operator (KNO), a generalization of the Fourier neural operator that allows for greater flexibility in kernel choices and for local spatial adaptivity while inherently using far fewer trainable parameters; (2) the ensemble DeepONet, a generalization to Deep Operator Networks that enables the incorporation of spatial adaptivity directly into a set of basis functions; and (3) a new operator learning paradigm based on kernel approximation that analytically preserves the divergence free property and requires minimal training, all while achieving state-of-the-art performance on incompressible flow problems.
We argue that operator learning has the potential to positively impact astrophysics through trustworthy, rapid, and interpretable surrogate models for multiscale simulations of magnetohydrodynamics (MHD) and numerical general relativity (GR), and for inverse problems such as physical parameter estimation.
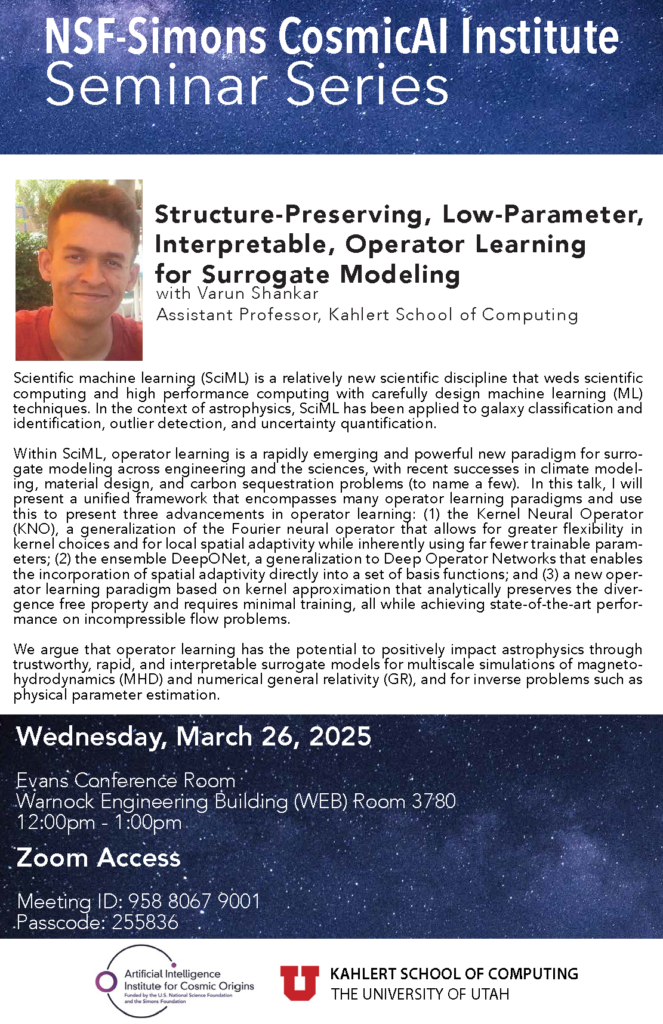
Goldman Sachs: Leadership and Economic Insights with Rob Kaplan - March 24
Event Information
March 24, 2025
2:00 PM - 3:00 PM
Rick and Marian Warner Auditorium in the Robert H. and Katharine B. Garff Building
____
Join Goldman Sachs on Monday, March 24 to hear unique insights on leadership and the economy from Rob Kaplan - Vice Chairman of Goldman Sachs and former CEO of the Federal Reserve Bank of Dallas.
Goldman Sachs is where exceptional people build extraordinary careers. We hire people with diverse skill sets, interests, and backgrounds - and we provide them with the hands-on experience to business challenges and opportunities to learn firsthand from the very best.
Register through the University of Utah Handshake page here.
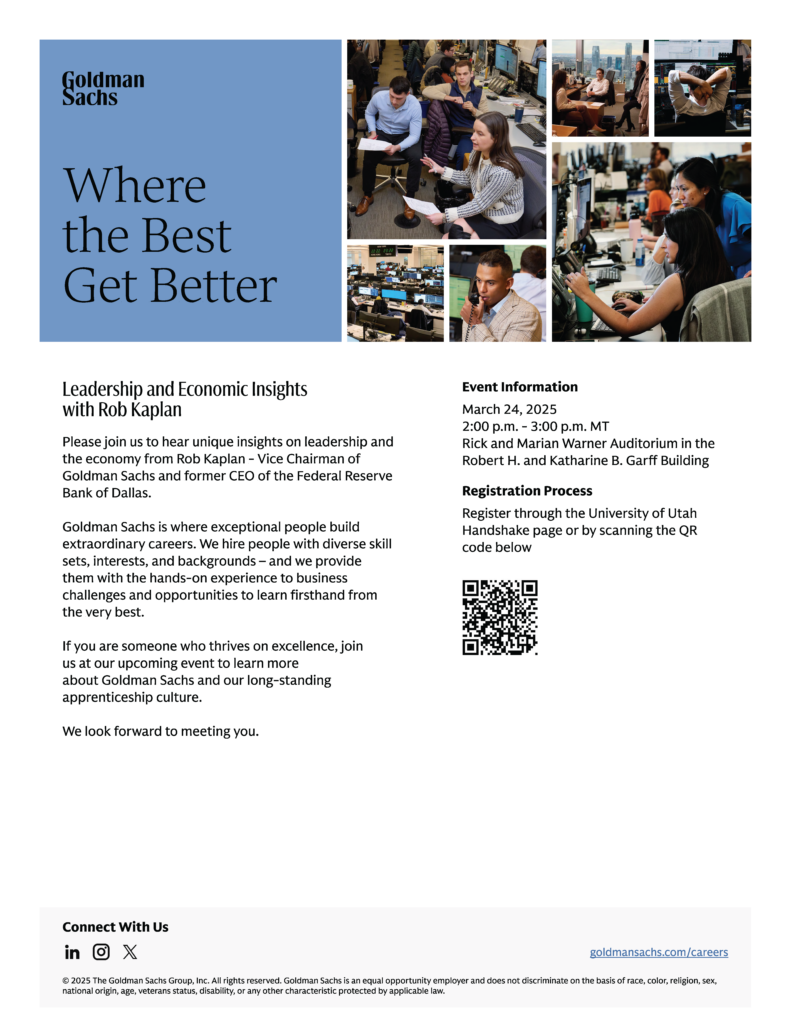
Announcing the Inaugural Kahlert Impact Prize Honorees
We are proud to recognize the inaugural recipients of the Kahlert Impact Prize.
The Kahlert School of Computing offers the Kahlert Impact Prize to two graduate students who, whether through research or service, show a track record of success and a compelling story of the high impact of their work. Honorees receive a scholarship of $2,000 each.
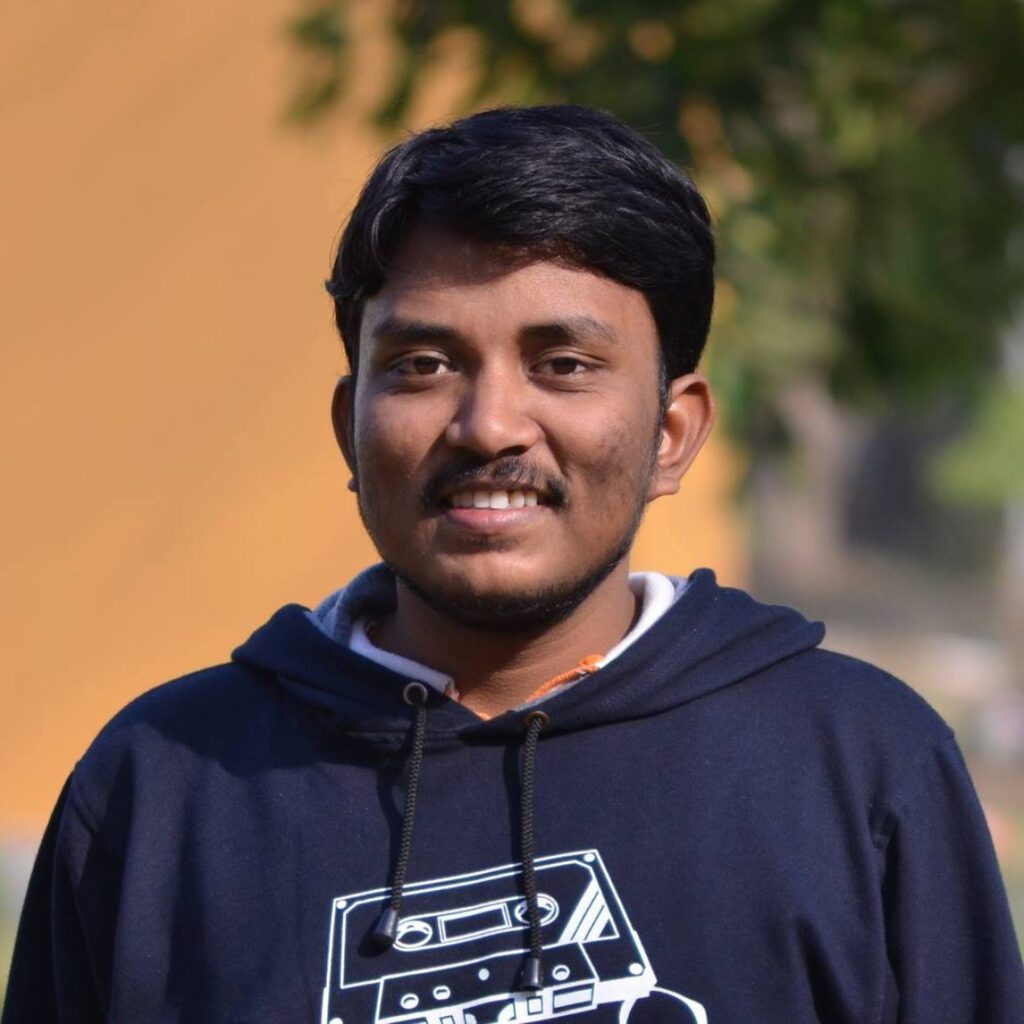
Amit Samanta
PhD Student
Amit works in the area of system design and implementation. His recent work has focused on serverless computing platforms, which are often deployed by large cloud services. Cloud workloads demand massive resources, and they are often dynamic and unpredictable. Amit’s contributions improve resource utilization while targeting performance and sustainability metrics.
Amit has published many papers at top systems conferences, earning him multiple awards. He has collaborated with industry professionals on some of his work. A key novelty is the deployment of scheduling algorithms that consider cutting-edge technologies like persistent or disaggregated memory. More recently, the carbon footprint of cloud platforms has come under scrutiny – Amit’s ongoing work explores carbon-aware and sustainability-aware network routing schemes. Amit has a long track record of service to his research community, including engagement in program committees, artifact evaluation committees, and event organizing.
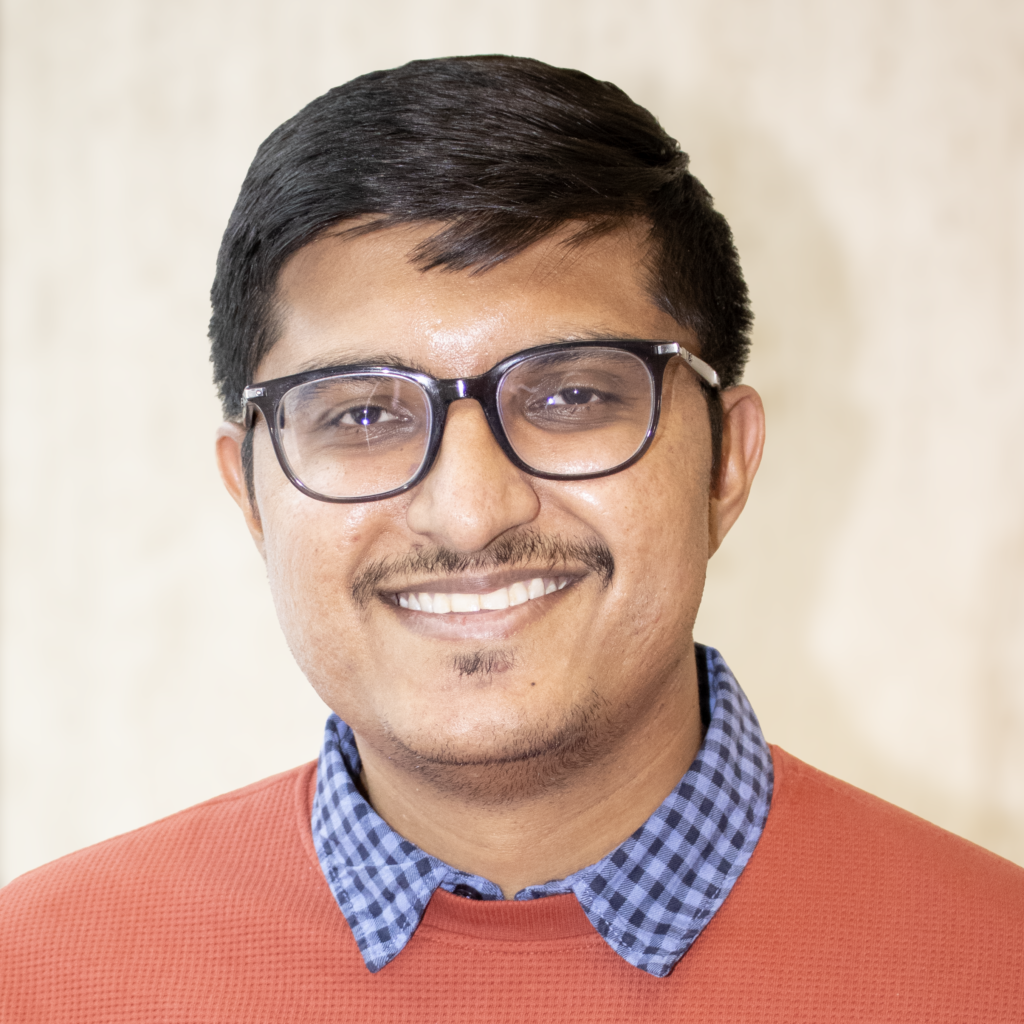
Maitrey Mehta
PhD Candidate
Maitrey works to expand the impact of AI to low-resourced languages. While most recent large language model advancements (like ChatGPT) are evident for English, progress in other languages has languished. Maitrey has focused on his native language of Gujarati, with hopes that it provides a roadmap to extend AI technologies to the many other languages spoken by the world’s population.
Maitrey’s vision is to give every human the right to interact with technology in one’s native language. To achieve this, he focuses on a key ingredient for developing this technology: data. Data is the fuel that powers modern LLMs, and there is an unfortunate data disparity across languages. His research aims to find efficient methods to close this resource gap. Maitrey contributed the first semantically annotated dataset in the Gujarati language that also captures cultural nuances. Subsequently, this dataset has been used to create dependency treebanks and other basic language tools like parsers and taggers. Maitrey has collaborated with industry and other groups on campus. He has helped the research community by serving on program committees and through mentorship roles. Among many talks on AI, he has also presented to an audience of veteran business owners at the 7th Annual Utah Veteran Business Conference in 2023.
UCBPC Computing Industry Panel—February 11
Tomorrow (February 11) at 3:30 PM, UCBPC hosts their Computing Industry Panel in Marriott Library Room 1120. Join us for an engaging discussion with professionals from top tech companies and academia. We hope to see you there!
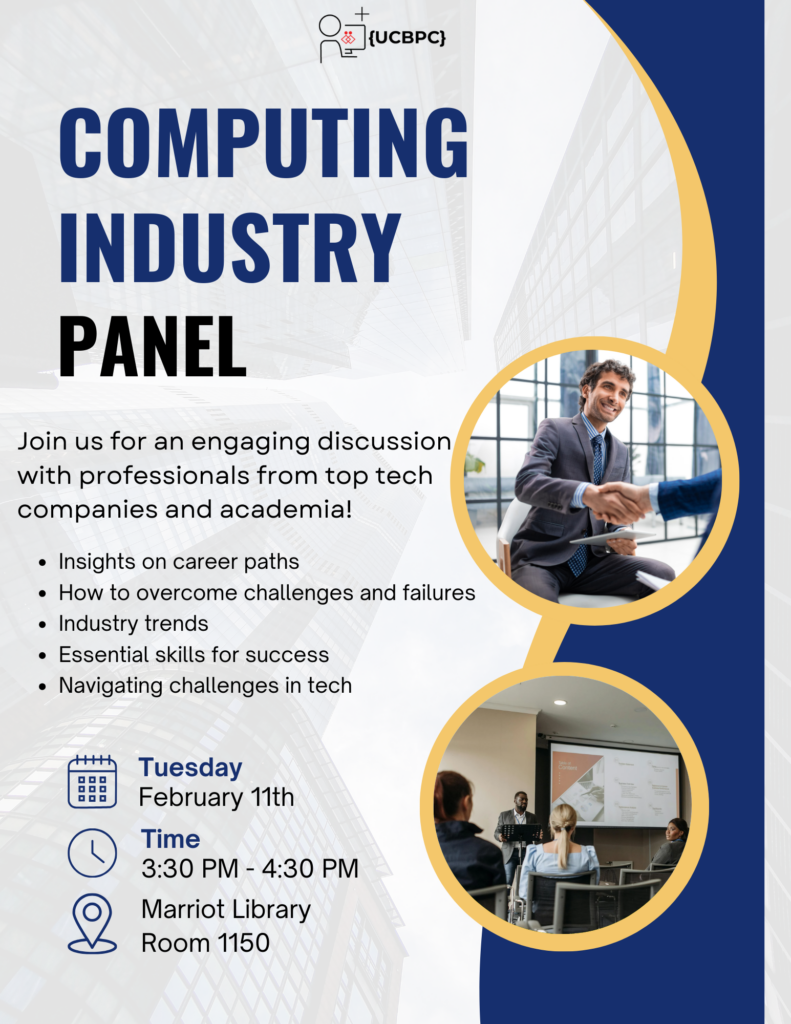
Distinguished Discussion Series with Don Norman—Thursday, February 27
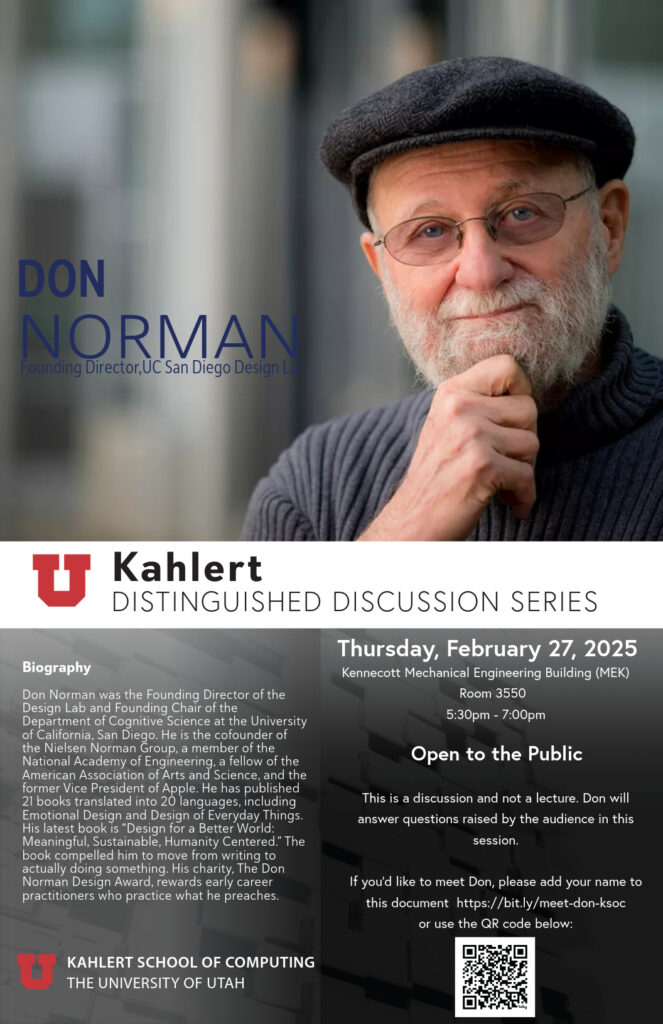
Join us Thursday, February 27, in the Kennecott Mechanical Engineering Building (MEK) 3550 at 5:30 PM for our Distinguished Discussion Series with Don Norman.
Don Norman was the Founding Director of the Design Lab and Founding Chair of the Department of Cognitive Science at the University of California, San Diego. He is the co-founder of the Nielsen Norman Group, a member of the National Academy of Engineering, a fellow of the American Association of Arts and Science, and the former Vice President of Apple. He has published 21 books translated into 20 languages, including Emotional Design and Design of Everyday Things. His latest book is Design for a Better World: Meaningful, Sustainable, Humanity Centered. The book compelled him to move from writing to actually doing something. His charity, The Don Norman Design Award, rewards early career practitioners who practice what he preaches.
This is a discussion and not a lecture. Don will answer questions raised by the audience in this session. The discussion is open to the public.
If you'd like to meet Don, please add your name to the document here.
2025 Data Science & AI Day Sees Over 200 Attendees, Keynote Speaker from Nvidia
Friday, January 24, in the A. Ray Olpin Student Union Ballroom. 229 students, faculty members, University staff members, and attendees from the general public gathered in the A. Ray Olpin Student Union ballroom on Friday, January 24, for The Utah Center for Data Science’s annual Data Science & AI day.
Keeping Us Posted
The event began with 10 research poster presentations from current students sharing their knowledge with peers and professors alike. Simultaneously, partners with University Career Success hosted a Data Science & AI Career Fair, connecting students with professionals across the Silicon Slopes as they prepare to enter the workforce.
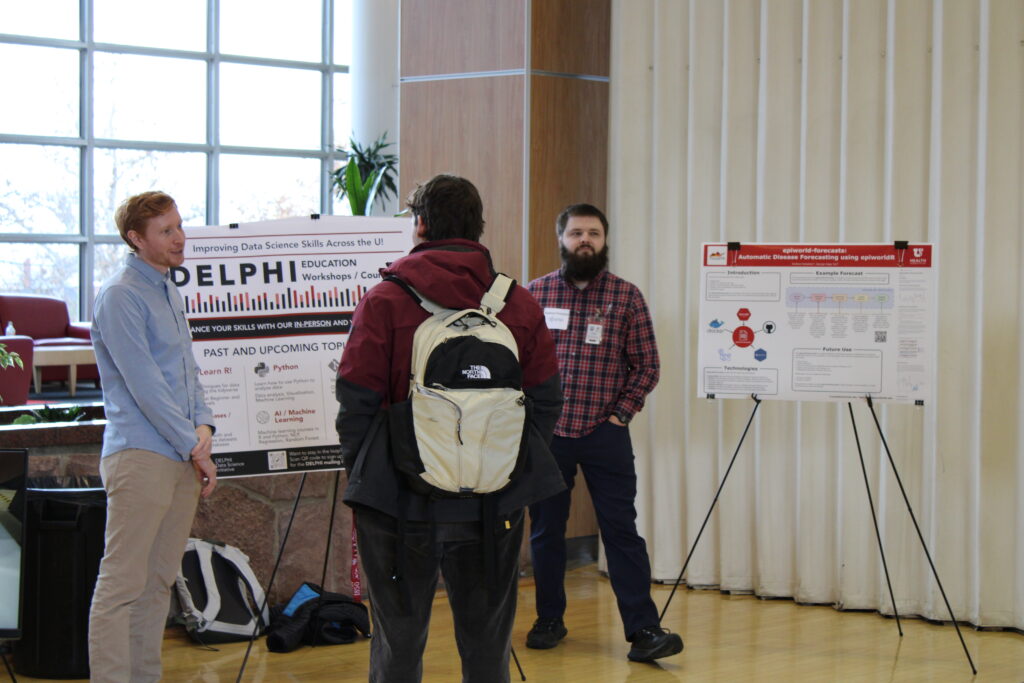
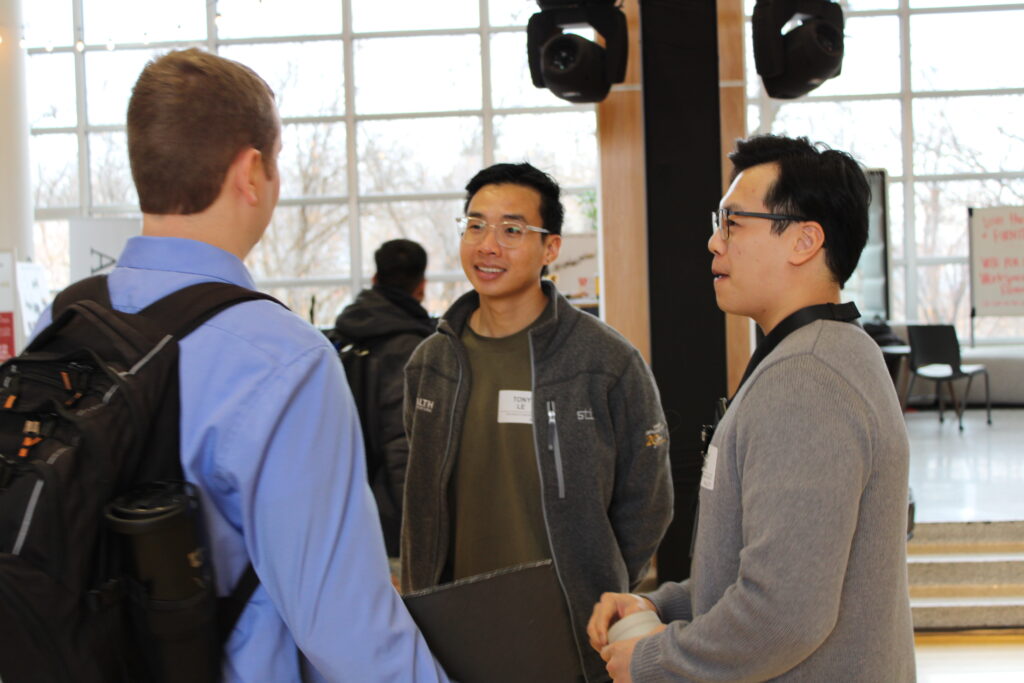
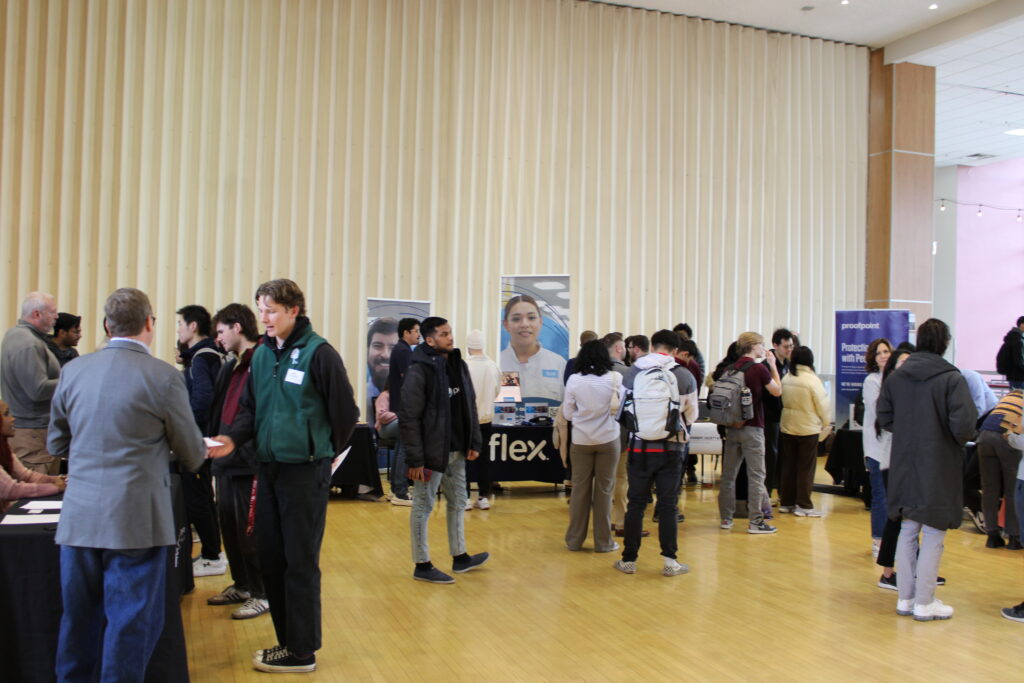
Keynote Speaker: Professor Dieter Fox of Nvidia & U Washington
The public has witnessed huge advances in generative artificial intelligence, including large language models, chatbots, and image and video generation tools. How has this progress impacted robotics?
This is the question that Dieter Fox, Senior Director of Robotics Research at Nvidia and University of Washington Professor, captivated the attendees while addressing during his keynote. Professor Fox identified large scale data as a primary ingredient to recent advances in generative AI. He then proposed several directions for generating large scale data for robot learning, focusing on his work in using large scale, parallelized simulation as the primary tool to enable massive data generation. He also discussed how this could be combined with human demonstrations. His keynote concluded by demonstrating exciting recent advances achieved using these techniques and gave his thoughts on how neural network architectures should evolve to further their use in robotics.
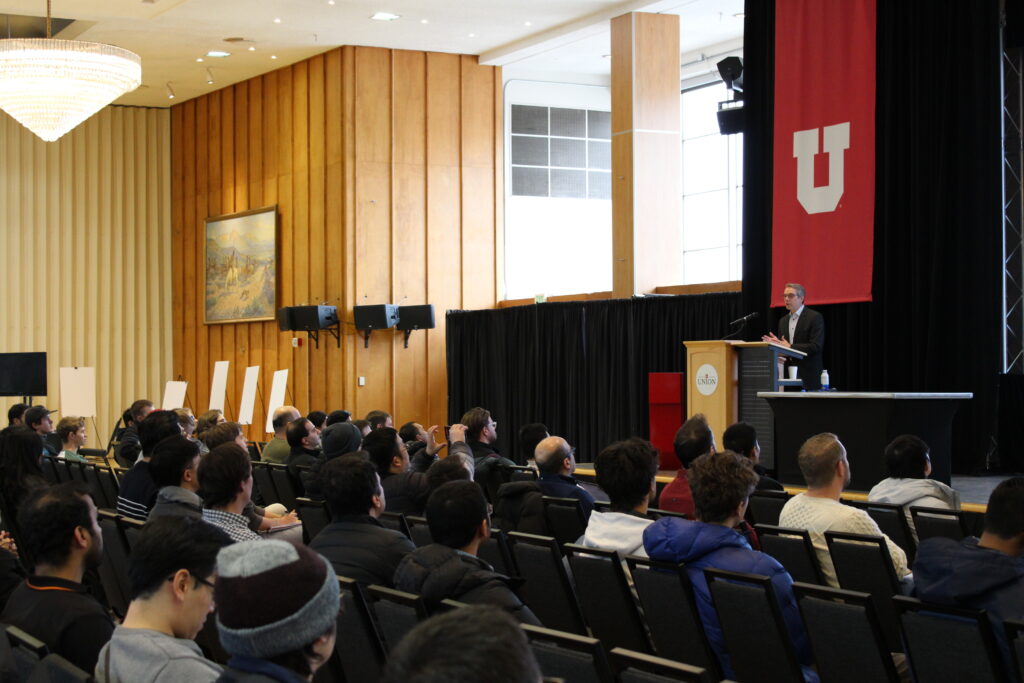
Research Highlights
The afternoon session culminated with research highlights from across the University who have practically applied data science & AI within their work.
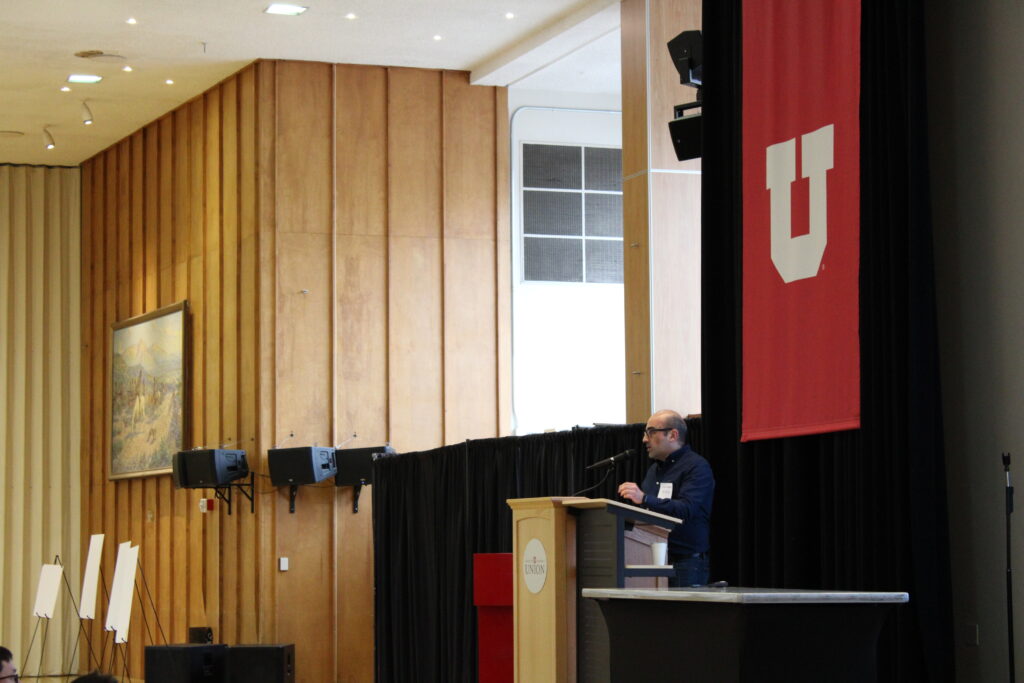
Assistant Professor Ziad Al-Halah's (Kahlert School of Computing) section "AI in Computer Vision" regarded spatial features in the audio-visual medium.
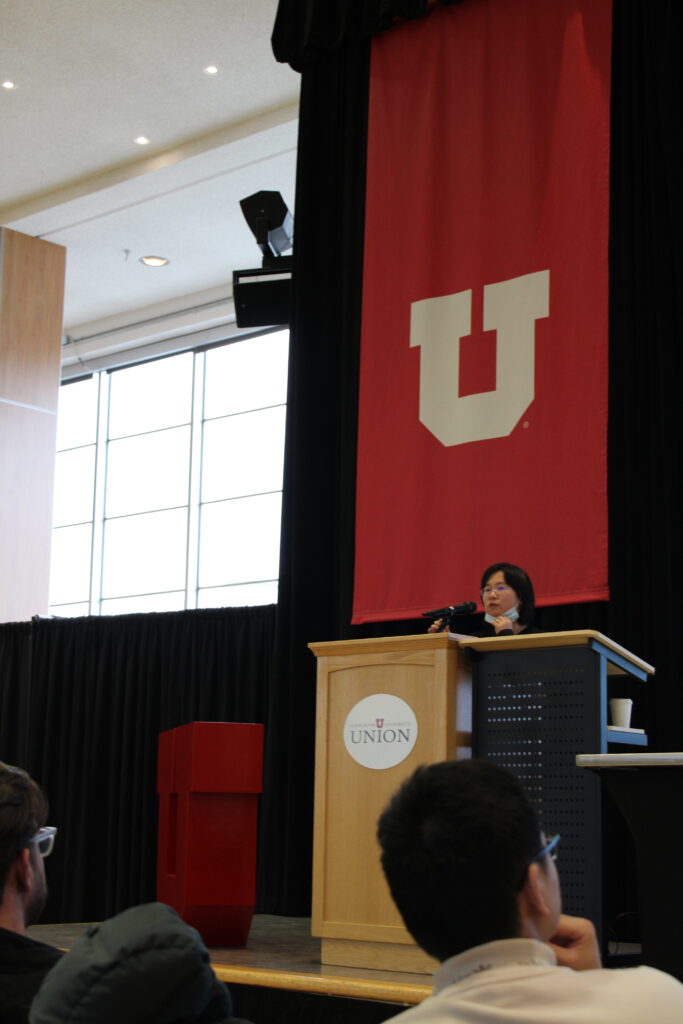
Associate Professor Xiaoyue Cathy Liu's (Civil & Environmental Engineering) section "DS of Human Mobility" discussed AI applications for traffic patterns and safety.
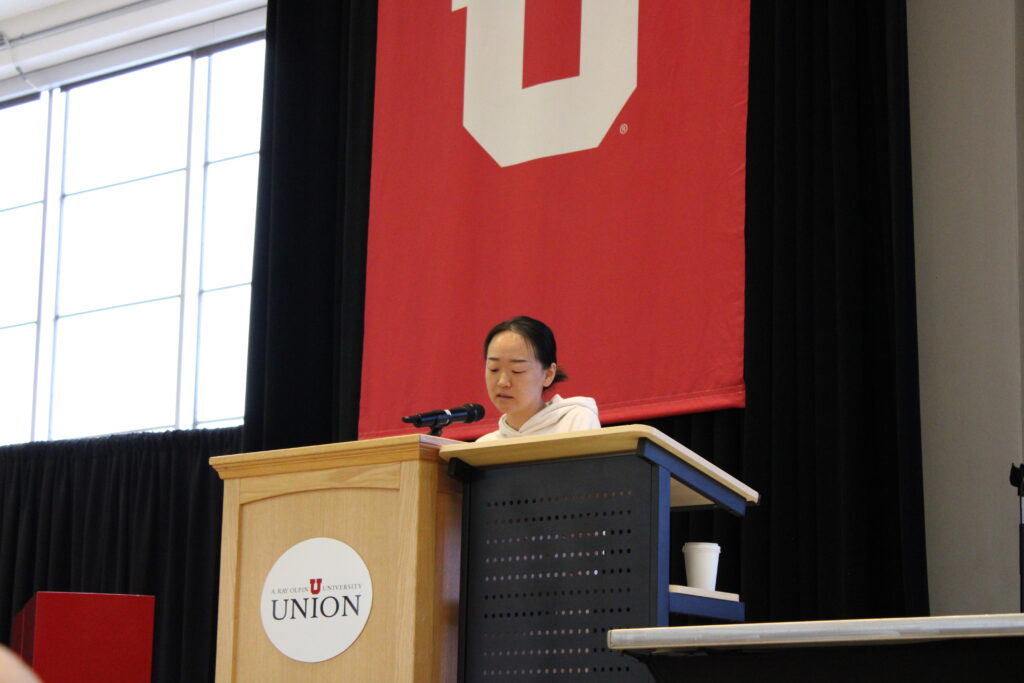
Research Assistant Professor Shiqi Yu (Physics and Astronomy) closed out the day's presentations with her "ML for Astronomy" section.
Guest Speaker: Proximal Causal Inference with Text Data talk by Katie Keith
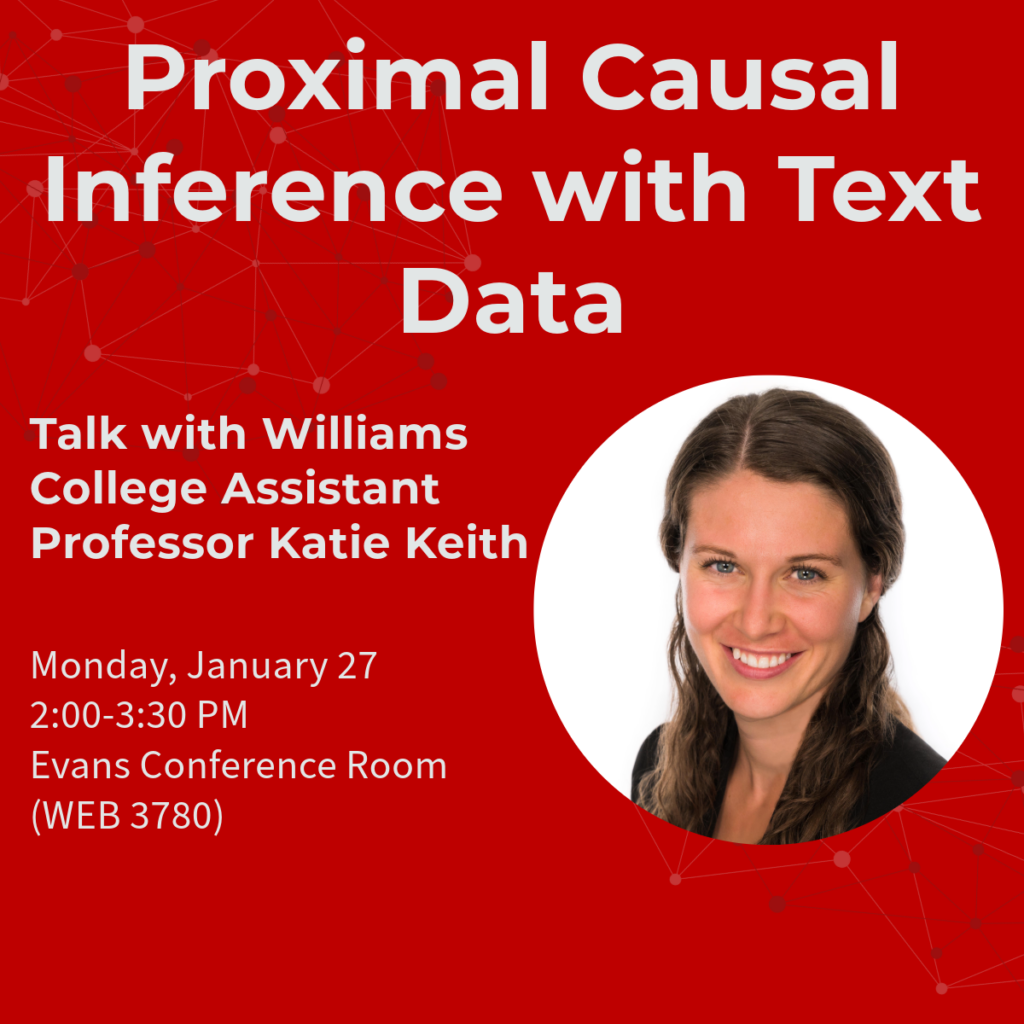
When: Monday, January 27, 2–3:30pm
Where: WEB 3780 (Evans Conference Room)
Talk Title: Proximal Causal Inference with Text Data
Speaker’s Bio: Katherine (Katie) Keith is currently an Assistant Professor in the Computer Science department at Williams College in Massachusetts. Her research interests are at the intersection of natural language processing, causal inference, and computational social science. Previously, she was a Postdoctoral Young Investigator at the Allen Institute for Artificial Intelligence, and she graduated with a PhD from the Manning College of Information and Computer Sciences at the University of Massachusetts Amherst. She has been a co-organizer of the First Workshop on Causal Inference and NLP, co-organizer of the NLP+CSS Workshops at EMNLP at NAACL, and was a recipient of a Bloomberg Data Science PhD fellowship.
Talk Abstract:
Causal inference underlies many important policy decisions and interventions. For example, clinicians must decide how to prescribe medications to patients, central bank committees must decide how to change interest rates, and online platform administrators must decide how to moderate users. In the absence of a randomized controlled trial, one can turn to observational (non-experimental) data to estimate causal effects. In this setting, a primary obstacle to unbiased causal effect estimation is confounding variables, variables that affect both the treatment (e.g., which medication) and the outcome (e.g., an aspect of patient health). In many applications, a rich, unstructured source of confounding variables is text data: notes from electronic health records (EHRs) detail patients’ personal and medical histories, newspaper articles document national and international events, and online platforms host exchanges of users’ written opinions. By expanding observational causal estimation methods that can incorporate natural language processing (NLP) and text data, analysts may be able to make inferences in a wider range of settings.
Recent text-based causal methods attempt to mitigate confounding bias by estimating proxies of confounding variables that are partially or imperfectly measured from unstructured text data. These approaches, however, assume analysts have supervised labels of the confounders given text for a subset of instances, a constraint that is sometimes infeasible due to data privacy or annotation costs. In this work, we address settings in which an important confounding variable is completely unobserved. We propose a new causal inference method that uses two instances of pre-treatment text data, infers two proxies using two zero-shot models on the separate instances, and applies these proxies in the proximal g-formula. We prove, under certain assumptions about the instances of text and accuracy of the zero-shot predictions, that our method of inferring text-based proxies satisfies identification conditions of the proximal g-formula while other seemingly reasonable proposals do not. To address untestable assumptions associated with our method and the proximal g-formula, we further propose an odds ratio falsification heuristic that flags when to proceed with downstream effect estimation using the inferred proxies. We evaluate our method in synthetic and semi-synthetic settings—the latter with real-world clinical notes from MIMIC-III and open large language models for zero-shot prediction—and find that our method produces estimates with low bias. We believe that this text-based design of proxies allows for the use of proximal causal inference in a wider range of scenarios, particularly those for which obtaining suitable proxies from structured data is difficult.