In September 2019, Jordan Berg, then a PhD student in the lab of Professor Jared Rutter in the Biochemistry Department, contacted Professor Bei Wang Phillips in the Kahlert School of Computing and the Scientific Computing and Imaging Institute regarding how best to design a tool for the analysis and visualization of metabolic networks. Over the next four years, then PhD student Youjia Zhou from Professor Wang Phillips’ lab collaborated with Jordan and Professor Rutter’s team. The collaboration has contributed toward two recent prestigious publications: one in Nature Cell Biology led by Jordan (Jordan A. Berg et al., 2023) and another in Science led by Dr. Kevin G. Hicks (Kevin G. Hicks et al., 2023). Berg and Zhou defended their PhDs in the Springs of 2022 and 2023, respectively. Dr. Zhou’s PhD thesis is titled “Topology-Based Visualization of Graphs and Hypergraphs.”
Metabolism is an important process in our bodies that affects various aspects of our health, such as cell growth and development, stress response, and energy production. It is a complex, interdependent network, and small changes in one area can have widespread effects throughout the entire metabolic system. Existing tools for analyzing metabolic networks suffer from various problems: they may be outdated, have limited functionality, be too technical to run for a biochemist, or completely unusable. To address these shortcomings, Zhou and Wang Phillips collaborated with Berg and Rutter’s team to develop new tools that leverage interactive graph visualizations. These tools are designed to analyze complex metabolic networks with a focus on detecting patterns and studying interactions between proteins and metabolites.
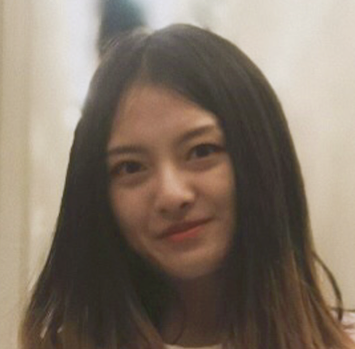
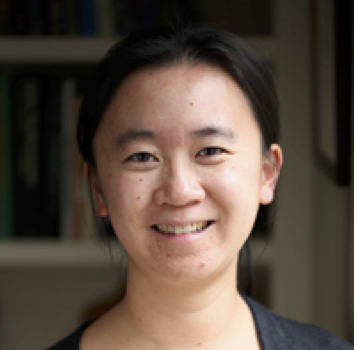
“Our vision is to develop practical visualizations of networks that help domain scientists with their knowledge discovery process,” said Professor Wang Phillips.
Pattern Recognition
The first interactive tool is called Metaboverse, a user-friendly tool that helps with the exploration of metabolic networks. In particular, it enables pattern recognition from a large, varied library of possible metabolic patterns. In the context of metabolism, a reaction pattern refers to a metabolic reaction that shows some change between the inputs and outputs of the reaction. Metaboverse enhances the understanding of detected patterns by providing a dynamic exploratory interface, which helps domain scientists come up with new ideas.
Previously, scientists searched for desired patterns manually, which was time-consuming and incomplete. Similarly, other available tools could find and identify only a few types of patterns and had the potential to overlook crucial insights. Metaboverse helps solve these problems: it introduces a broad collection of patterns and, using automated techniques, provides a quick and accurate way for scientists to detect complex patterns that they might have previously missed.
In terms of practical applications, Metaboverse was used to find patterns in the metabolism of early-stage human lung adenocarcinomas (LUAD), a type of lung cancer. The goal was to identify which metabolites could be used to diagnose the disease at an early stage. Metaboverse was able to prioritize patterns in nucleotide metabolism reliably; consistent with the original study as well as the manual re-analysis of the data using other existing tools. In addition, Metaboverse helped discover new patterns related to xanthine metabolism and a group of reactions linked to a decrease in lysine. These discoveries were made using data from a previous study where changes in these metabolites were observed but not fully explored.
Analysis of Protein-Metabolite Interactions
Protein-metabolite Interactions (PMIs) indicate how proteins and metabolites communicate metabolic status to different cellular processes. In metabolic networks, finding PMIs that mediate these networks can be difficult because they often have low affinity. To help solve this problem, Zhou and Wang Phillips worked with Berg and Rutter’s team to create MIDAS, a library that helps find PMIs systematically. Specifically, the researchers helped develop Electrum, a flexible, user-friendly visualization portal that aids in the understanding and analysis of the PMI data generated by MIDAS. While other general visualization platforms are unable to handle PMI data because of the specific analytical capabilities it requires, Electrum can differentiate between known and unknown PMIs, which can lead to new discoveries.
The usefulness of Electrum was demonstrated through a case study in which interactions within and between pathways in carbohydrate metabolism were discovered. Carbohydrate metabolism enzymes drive most of the energy production and biosynthetic precursor generation of cells, and their regulation involves metabolite interactions. The MIDAS platform identified 830 putative PMIs, many of which were previously unknown. Electrum provided interactive visualizations to help domain scientists comprehend the PMIs within and between pathways, and the visual analytics results revealed regulation within and between pathways in carbohydrate metabolism. The collaboration led to a recent publication in Science led by Dr. Kevin G. Hicks.
In the future, the researchers will continue developing and enhancing interactive visualization tools to help domain scientists better explore and understand complex networks.